Identity Fraud Accelerates in 2020
The year 2020 was a heyday for online fraudsters. There are a couple of reasons why. First, consumers were forced from a digital-first to a digital-only business environment, creating an exponential surge in data. Second, the U.S. government distributed more than $1 trillion in economic stimulus for struggling families and businesses as a result of the COVID-19 pandemic. Fraudsters saw this as an opportunity to exploit programs like the Payroll Protection Program and expanded unemployment insurance.
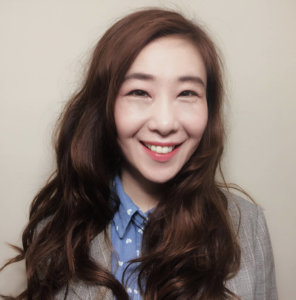
“When we track the fraud trends in our own data, we see that the authorized user abuse risk in 2020 went up by over 23% compared to 2019 and 2018,” said Cori Shen, leader of the Identity and Fraud Data Science Team at Equifax. Cori and I discussed what can be done to mitigate these threats during the Data Dialogues podcast episode, Using Smart Data to Combat Identity Fraud. “These fraud schemes might be new,” she added, “but the underlying fraud challenges are the same ones... like synthetic identities and compromised identities, which have been around for years.”
“We Want Data to Talk”
So, what can organizations do to combat these challenges? The answer lies in leveraging data and analytics to achieve what Cori calls smart data. “What matters most is how to make sense of big data and how to intelligently and efficiently assemble multi-source data for the right insights. And we will call it smart data because we want data to talk, and we want data to be able to offer recommendations,” she added. Listen to our podcast now, especially if you’re a data scientist, to understand how smart data can be both predictive and prescriptive.
Below is an excerpt from the podcast.
Smart Data has Two Components
Sheth: I love it. Smart data. I mean, it sounds fantastic, right? But it's easier said than done, isn't it? Let's take synthetic identities for example. We know that many of these have been in the system for a while, and they look like legitimate people. Very often their identity information is complete, and it matches to what systems have. As a matter of fact, sometimes they even have a matched social media profile. That's why these fake identities look like real people and can be used to create fake businesses, defraud the system with millions of dollars of PPP or employment claims. Right? So even if we do identity verification matches from multiple sources, we may not be able to catch them. So what should we do?
Shen: If we're just talking about matching identities from multiple sources, it is not smart data.
Smart data has two components: insights and connections. We think a real effective way to build smart data is to connect to the useful insights from a graph network perspective.
Let me take synthetic ID detection for example. Here is how you can build. First, build useful insights from multiple sources. You want to search for the abnormal signals throughout an identity's lifecycle. To do so you will need the consumer activity data from multiple sources and from multiple systems.
For example, the consumer applies for credit cards or loans. The consumer checks their credit online. They enroll. We're logging into an online system. They're making payments. They're making purchases from e-commerce sites. All these different data points are consumer activity data. When you get the power of the consumer activity data, you can look closely into their activities. And then, you will find out a lot of secrets about them. Here is an example. All the synthetic ID outliers appear at an early stage. You will see some synthetic IDs apply for mortgages and shop for luxury cars.
However, when you look at the activity pattern for a regular legit consumer at the earlier stage, you will often see they only apply for cell phone, apartments, internet service, credit cards. These types of starter programs.
Sheth: So what about the digital signals and bureau data? I would think they are also very useful in identifying synthetic identities, right?
Shen: Yes, digital signals are definitely powerful and critical. Here's another example of synthetic ID.
To establish and maintain synthetic IDs, the fraudsters like to manipulate identities via online channels. They like to change addresses and alter names online or from their mobile phones. At that time, you may see there could be the same device links to many different IDs for name and address change requests. You may also see that the IP geolocation is far away from the existing addresses they're using and the new addresses they requested. Speaking of bureau data, it is also really helpful when you use them to explore the risk of signals like piggybacking credit using authorized user abuse schemes.
Listen to the full episode for more on how the data science lab at Equifax processes billions of records to make prescriptive and predictive insights that help fight identity fraud. Don’t miss the other episodes in our Data Dialogues series. You can also learn more about the series on our website.