How Better Attributes Result in More Predictive Analytics
It All Starts with Building the Right Attributes
With the data explosion we’ve seen in recent years, businesses have access to more data than they’ve ever had before. It’s important for their data scientists to understand what data to use and how to use it -- all within a more efficient timeframe. Once companies have tapped into the right mix of data sources, they still need an efficient testing and production process. This will help deploy analytics faster in order to deliver more customer-focused products and services. It all starts with building the right attributes – the building blocks of models and strategies that help improve predictiveness and performance.
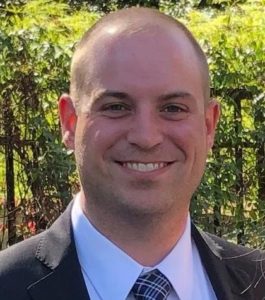
“Think of attributes as the representation of data that businesses feed into a model or decision strategy,” says Ryan Baltes, Analytics Product Manager, Equifax. “When dealing with attributes in data, companies need to be able to access this information quickly, and use them in production to build the decision strategies they need."
Move From Analytics to Production in Days -- Not Months
Traditionally, it would take quite a bit of time and effort for businesses to access new data sources and develop new attributes. With today's more advanced automation tools, data scientists can build, test, manage, and package attributes without having to recode them for use in production. This helps them move from analytics to production in a matter of days, not months.
"In today’s regulatory environment, there’s an increased focus on explainability and transparency,” says Baltes. “So you must be able to explain the attributes used in model development. Also, each new model or new data analyst added to the project produce more attributes. This can create what we call ‘attribute sprawl’. There are often regulatory requirements that must be met to manage the predictions being created from these multitudes of attributes. So there is a real need to manage the attribute and model lifecycle, and get a better handle on that sprawl.”
More efficient attribute development and deployment is possible. There are toolsets that can remove the recoding step, automatically generate documentation from scanning the code instead of interpreting manual documentation, find duplicate fields of information, and provide lineage analysis. Additionally, businesses can employ attributes that are transparent and consistent when they use an attribute management strategy backed by an automation tool. Additionally, built-in management features can allow other teams (such as Compliance, Legal, Audit, etc.) to have a view into attribute definitions and documentation.
Find out more on our website.