Credit Scoring Leaders Reveal Innovations in Data and Analytics
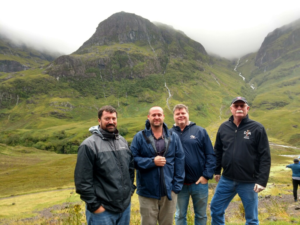
Last week, top data and analytics experts from Equifax and around the world convened in Edinburgh, Scotland for Credit Scoring and Credit Control XVI, Europe’s prestigious biennial conference for credit scoring and related topics. The Equifax team was honored to participate and had an overwhelming presence with 11 papers accepted, which was more than any other organization in attendance. In recent years, our advanced data and analytics group has focused on financial inclusion and creating more opportunities for more consumers.
The resulting new patents and new technologies in emerging areas of machine learning and explainable AI are helping to transform traditional credit scoring by making financial products more accessible to a wider audience of consumers. Additionally, they're helping businesses keep risk levels stable. These innovations and others were showcased in their conference papers and presentations. Here's a brief rundown of the 11 Equifax conference papers or you can view the full papers.
1. Explainable AI (xAI) - Predictive Models with Explanatory Concepts: A General Framework for Explaining Machine Learning Credit Risk Models That Simultaneously Increases Predictive Power
Presenters: Michael McBurnett, PhD and Matthew Turner, PhD This presentation describes a method of utilizing collinear attributes to increase the predictive power in a credit risk model while simultaneously generating model explanations. We make use of collinear attributes to construct a predictive model. The full predictive power of a modeling technique is realized by not reducing the dimension of the predictor space. Factor analysis is then used to generate interpretable concepts that are extracted from the predictor space and used for model explanations. Predictive Models with Explanatory Concepts can be used for machine learning or artificial intelligence transparency requirements (i.e. explainable AI) imposed by business decision makers or regulators.
2: Explainable AI (xAI) - Approximating an Optimal Path to Improve a Consumer’s Credit Score
Presenters: Lewis Jordan, Allan Joshua, Stephen Miller, PhD and Matthew Turner, PhD With the advent of the General Data Protection Regulation and advice from UK regulators, including the Information Commissioner’s Office, there's an increasing expectation on financial institutions. By using consumer data for credit risk applications, they have to explain how automated systems make decisions. As a result, this increases financial transparency and makes information more accessible to consumers. However, explanations of credit risk models do not always translate into the ordered sequence of actions a consumer could take to improve their score to a desired threshold, except under strict assumptions. Further, the use of machine learning models complicates transforming model explanations into actionable consumer behavior due to the inherent nonlinearity and interactions present in these models. In this work, we describe a method for constructing an optimal path for an individual consumer that explicitly helps each navigate the model feature space to a desired score. As opposed to currently available score simulators, our methodology automatically generates the sequence of actions a consumer could take to reach a specified credit score.
3: Explainable AI (xAI) - Direct Estimation of the Survival Function for Credit Default Using Nested Time Intervals
Presenter: Jeffery Dugger, Michael McBurnett, PhD Traditional credit risk models focus on predicting the probability that an account will default in a defined performance window, typically 24 months. This paper focuses on predicting the probability of when an account will default in the performance window. Knowing when a borrower is likely to default allows lenders to price term loans more accurately, as well as help them predict time between defaults for a given customer, so they can better manage portfolio risk. Survival analysis predicts the probability of when an event will occur by using three interrelated functions: the survival function, the hazard function, and the probability function. The survival function predicts the probability an account will remain good up to a given time, the hazard function provides the default rate over time, and the probability function shows the distribution of default times.
4: Explainable AI in Practice: Using NDT Gen 3 with UK CRA Data
Presenters: Stephen Miller, PhD, Kamini Patel, Steven Upton, Trang Luong, Natalie Scott, Tanvi Verma NeuroDecision® Technology(NDT) is an Equifax-patented explainable AI solution based on monotonically constrained neural networks. This presentation shares our experience using NDT Gen 3 with UK CRA data including:
- Applying constraints to non-monotonic, categorical and ordinal characteristics
- Handling default (special non-numeric) values
- Model performance compared to traditional scorecards, vanilla logistic regression and unconstrained neural networks
- Transparency and explainability of individual scores/decisions
5: Transactional Data and Credit Risk
Presenters: Steven Baker, Daniel Weaver, Harvey Lawrence from Account Score This presentation explores the use of transactional data for predicting credit risk with a focus on the analytics and techniques that make this possible, where transactional data adds most value and how together transactional data and traditional CRA data can power better lending decisions. Key points addressed include:
- A comparison of transaction data versus traditional CRA data in assessing credit risk
- The benefits of combining CRA data and transactional data analytics together to split risk more powerfully
- How transactional data can transform the treatment of consumers with “thin” credit files
- The analytical techniques used to drive the most value from the granular data
- Insights into specific segments and transactional features that are highly predictive and the rationale behind them
6: Affordability – Past, Present and Future
Presenters: Steven Baker, Alice Zanotti, Daniel Weaver Open banking can reduce the time taken to source bank statements as part of a mortgage application from weeks to minutes. It enables the secure and digital transfer of a consumer’s bank statement and provides consumers with a faster and smoother customer journey. This presentation explores the next stage of the application process. What do lenders do when they receive the bank transaction data? How do they assess and automate affordability assessments using this new data? How does this new assessment compare versus current methods of evaluating affordability? Based on analytical research and development undertaken on bank account transaction data, this presentation will address:
- How income is defined, by the regulator, lenders and the consumer. Are we all talking the same language?
- Comparison of different methods of verifying income available to lenders today, from manual to automated, current account turnover to open banking
- Looking beyond salary, what are the other components of income? And how do you identify them in transaction data?
- Assessment of the accuracy of different methods of estimating income
- Complications in calculating income from different datasets
- How predictive of credit risk is income?
- How consumer and household expenditures are calculated. Which expenditures can you obtain from bank account data? Which ones are you missing?
- Validation of expenditure estimates
- Does the sharing of transaction data through consent result in biased samples of income and expenditure?
7: Developing a Credit Risk Forecasting System for IFRS 9 and Stress Testing Using Industry and Pooled Cross-Section Data
Presenter: Vassilis Ioannou This presentation provides overviews of credit risk forecasting techniques, problems to capture the impact of economy, economic forecasting techniques and problems to use for credit risk purposes due to data limitations. In addition, it explores:
- A methodological approach to combine credit risk and economic forecasting methods, using industry and pooled cross-section data (i.e. Equifax Bureau) to address data limitations
- A performance evaluation of the proposed approach in the context of IFRS9 and stress testing
- Practical issues and operationalizing IFRS9 and stress testing calculations using Equifax Bureau
8: Long-run and Downturn Credit Risk Estimates for Basel IRB Using Bureau Data
Presenter: Vassilis Ioannou This presentation examines the Basel Credit Risk Model and the nature of the required estimates probability of default (PD) and loss given default (LGD) under the Basel Internal Ratings Based (IRB) approach. It also discusses evolving regulatory guidelines applicable to the calculation of long-run PD and downturn LGD, and explores:
- A methodological approach to estimate long-run and downturn credit risk estimates, using Industry and Pooled Cross-Section Data (i.e. Equifax Bureau) to address data limitations and reflect economic conditions since 1990
- A performance evaluation of the proposed approach
- Practical issues and regulatory landscape for using external data (including Equifax Bureau data) for IRB
9: Credit Scoring – Where is the Power?
Presenters: Steven Upton, Steve Baker, Natalie Scott With the growing maturity of open banking and the resurgence of machine learning techniques in the credit risk industry, the boundary of credit scoring power is once again expanding. This presentation reviews the fundamental components that can impact the predictive power of scores, and how these different components impact score stability over time. Utilizing the Bureaus unique data assets allows the testing of all elements of a development, with the unparalleled ability to explore concepts in depth and beyond the time horizons associated with a typical build. This presentation will cover such topics as:
- Sample design: exploring the impact of sample size, sampling methodology and weights.
- Data depth: what improvement does historical data add
- Features: exploring how the number, type of characteristics impact the score
- Segmentation: is there an optimal number of segments; when do the benefits of segmenting start to dwindle
- Observation window and seasonality and the outcome definition: does seasonality really affect score performance; what impact does the use of indeterminates have
- Statistical technique: how do machine-learning scores hold up over time, versus traditional scoring techniques
10: Credit Scoring - Reject Reference and Inference: The Power Behind Differing Methodologies
Presenters: Chantel Pistorius, Kathryn Somers When developing acquisition scorecards, it is always important to assess rejected applications. This allows you to correct for any potential bias in the accepted population. Additionally, you can ensure the model is aligned to a ‘through the door’ population. Several Reject Inference methods exist, but which is the most powerful and what other considerations need to be taken into account? This presentation reviews and assesses both Reject Reference and Reject Inference methodologies, evaluating the benefits of each and how this drives scorecard performance. Key points covered in this presentation include:
- Overview of the Reject Inference methodologies considered: parcelling, extrapolation etc.
- Penalty factor: how to select the most appropriate value
- Other considerations: understanding any other factors that can impact the selection of the best model
- Reject Reference vs Reject Inference
- Comparison results: a comparison of each methodology when performed on a data sample, including performance measures (e.g. Gini), pros and cons
11: Risk Assessment of Small Businesses - The Power of Commercial Credit Data Sharing
Presenters: Tony Mott, Zachary Harries Historically, credit reports for smaller businesses, especially those that are unincorporated, held limited financial information. This made the risk assessment of these entities a challenge. The advent of the Commercial Credit Data Sharing scheme[1](CCDS) means information on a business’ financial activity and health is more accessible than ever before. The amalgamation of current account information and the broad range of data facilitates a better assessment of the liquidity, financial commitment, behaviors and ability of a small business to repay a credit line. This presentation explores:
- How CCDS data helps support better lending decisions for small businesses
- Closing the knowledge gap
- And providing additional power over traditional commercial bureau assets